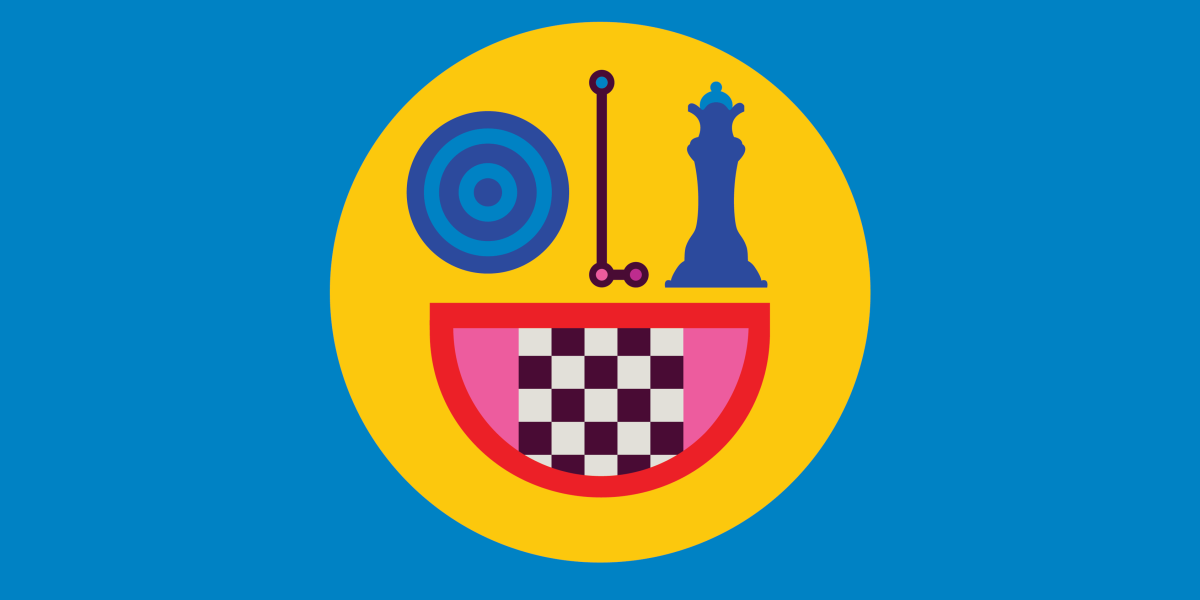
What the history of AI tells us about its future
But what desktops were being lousy at, ordinarily, was strategy—the capacity to ponder the condition of a activity lots of, quite a few moves in the long run. That’s the place human beings however had the edge.
Or so Kasparov thought, until eventually Deep Blue’s transfer in recreation 2 rattled him. It seemed so refined that Kasparov started worrying: probably the device was considerably far better than he’d believed! Certain he experienced no way to acquire, he resigned the next game.
But he should not have. Deep Blue, it turns out, was not in fact that excellent. Kasparov experienced failed to location a shift that would have permit the video game stop in a draw. He was psyching himself out: concerned that the equipment may well be significantly much more strong than it really was, he had started to see human-like reasoning exactly where none existed.
Knocked off his rhythm, Kasparov retained participating in even worse and even worse. He psyched himself out above and in excess of once again. Early in the sixth, winner-can take-all recreation, he produced a go so lousy that chess observers cried out in shock. “I was not in the mood of participating in at all,” he afterwards claimed at a push convention.
IBM benefited from its moonshot. In the push frenzy that adopted Deep Blue’s accomplishment, the company’s sector cap rose $11.4 billion in a solitary week. Even extra important, even though, was that IBM’s triumph felt like a thaw in the long AI wintertime. If chess could be conquered, what was upcoming? The public’s mind reeled.
“That,” Campbell tells me, “is what received folks paying interest.”
The fact is, it was not stunning that a computer conquer Kasparov. Most persons who’d been paying out consideration to AI—and to chess—expected it to occur sooner or later.
Chess may feel like the acme of human believed, but it is not. Without a doubt, it’s a mental job that is pretty amenable to brute-force computation: the principles are clear, there’s no hidden details, and a personal computer doesn’t even need to have to continue to keep track of what occurred in preceding moves. It just assesses the posture of the parts proper now.
“There are pretty few complications out there in which, as with chess, you have all the information you could possibly need to have to make the right determination.”
Everyone understood that the moment computers acquired speedy plenty of, they’d overwhelm a human. It was just a concern of when. By the mid-’90s, “the creating was previously on the wall, in a perception,” suggests Demis Hassabis, head of the AI firm DeepMind, element of Alphabet.
Deep Blue’s victory was the minute that showed just how confined hand-coded methods could be. IBM experienced spent many years and tens of millions of pounds producing a pc to engage in chess. But it could not do just about anything else.
“It did not lead to the breakthroughs that allowed the [Deep Blue] AI to have a enormous impression on the world,” Campbell states. They didn’t truly uncover any ideas of intelligence, simply because the genuine world doesn’t resemble chess. “There are extremely handful of challenges out there where, as with chess, you have all the information you could perhaps will need to make the ideal choice,” Campbell adds. “Most of the time there are unknowns. There is randomness.”
But even as Deep Blue was mopping the flooring with Kasparov, a handful of scrappy upstarts ended up tinkering with a radically more promising sort of AI: the neural web.
With neural nets, the notion was not, as with qualified systems, to patiently write procedures for each decision an AI will make. In its place, coaching and reinforcement fortify interior connections in rough emulation (as the principle goes) of how the human brain learns.
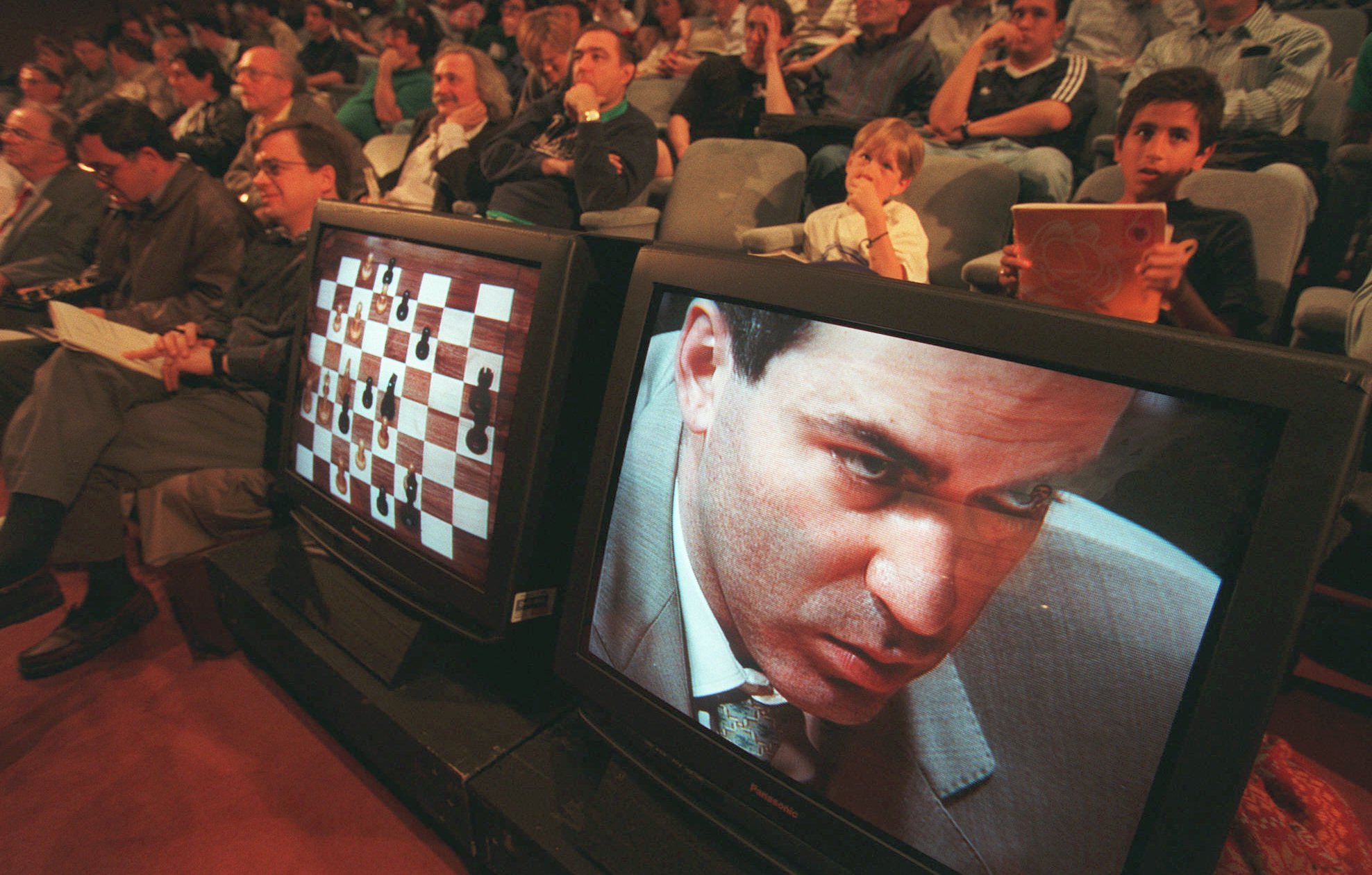
AP Photograph / ADAM NADEL
The concept experienced existed given that the ’50s. But instruction a usefully substantial neural internet necessary lightning-rapid computers, tons of memory, and tons of knowledge. None of that was easily accessible then. Even into the ’90s, neural nets ended up deemed a squander of time.
“Back then, most men and women in AI thought neural nets ended up just rubbish,” states Geoff Hinton, an emeritus pc science professor at the University of Toronto, and a pioneer in the discipline. “I was identified as a ‘true believer’”—not a compliment.
But by the 2000s, the laptop marketplace was evolving to make neural nets practical. Video-recreation players’ lust for at any time-far better graphics made a enormous sector in ultrafast graphic-processing models, which turned out to be beautifully suited for neural-web math. Meanwhile, the world-wide-web was exploding, making a torrent of pics and textual content that could be used to practice the programs.
By the early 2010s, these complex leaps were being letting Hinton and his crew of correct believers to acquire neural nets to new heights. They could now create networks with quite a few layers of neurons (which is what the “deep” in “deep learning” signifies). In 2012 his workforce handily gained the yearly Imagenet levels of competition, wherever AIs contend to identify components in photos. It surprised the entire world of laptop or computer science: self-finding out machines had been lastly practical.
Ten yrs into the deep-learning revolution, neural nets and their pattern-recognizing skills have colonized each individual nook of daily daily life. They assist Gmail autocomplete your sentences, assistance financial institutions detect fraud, permit photo apps mechanically realize faces, and—in the scenario of OpenAI’s GPT-3 and DeepMind’s Gopher—write extended, human-sounding essays and summarize texts. They are even switching how science is performed in 2020, DeepMind debuted AlphaFold2, an AI that can forecast how proteins will fold—a superhuman talent that can assist information researchers to acquire new prescription drugs and treatments.
Meanwhile Deep Blue vanished, leaving no handy innovations in its wake. Chess participating in, it turns out, was not a laptop skill that was required in each day life. “What Deep Blue in the close confirmed was the shortcomings of trying to handcraft everything,” says DeepMind founder Hassabis.
IBM attempted to remedy the predicament with Watson, a further specialised technique, this one particular built to deal with a a lot more simple challenge: acquiring a device to reply inquiries. It utilised statistical investigation of substantial amounts of textual content to attain language comprehension that was, for its time, chopping-edge. It was much more than a straightforward if-then procedure. But Watson confronted unlucky timing: it was eclipsed only a handful of years later on by the revolution in deep mastering, which brought in a era of language-crunching designs much more nuanced than Watson’s statistical techniques.
Deep finding out has run roughshod around outdated-faculty AI exactly due to the fact “pattern recognition is exceptionally impressive,” states Daphne Koller, a former Stanford professor who founded and operates Insitro, which uses neural nets and other forms of device finding out to investigate novel drug treatment plans. The adaptability of neural nets—the extensive wide variety of techniques sample recognition can be used—is the rationale there has not but been a further AI wintertime. “Machine understanding has truly sent price,” she suggests, which is a little something the “previous waves of exuberance” in AI never ever did.
The inverted fortunes of Deep Blue and neural nets exhibit how negative we were being, for so prolonged, at judging what’s hard—and what is valuable—in AI.
For decades, folks assumed mastering chess would be essential because, very well, chess is tricky for human beings to play at a superior stage. But chess turned out to be relatively uncomplicated for computers to master, for the reason that it’s so logical.
What was considerably harder for desktops to learn was the informal, unconscious mental get the job done that human beings do—like conducting a lively discussion, piloting a vehicle as a result of targeted visitors, or examining the emotional condition of a friend. We do these points so effortlessly that we seldom recognize how tricky they are, and how much fuzzy, grayscale judgment they require. Deep learning’s good utility has occur from becoming capable to seize small bits of this delicate, unheralded human intelligence.
Nevertheless, there’s no final victory in artificial intelligence. Deep studying may perhaps be using significant now—but it’s amassing sharp critiques, much too.
“For a really lengthy time, there was this techno-chauvinist enthusiasm that all right, AI is going to clear up just about every difficulty!” suggests Meredith Broussard, a programmer turned journalism professor at New York College and writer of Artificial Unintelligence. But as she and other critics have pointed out, deep-discovering techniques are normally skilled on biased data—and take up these biases. The laptop or computer researchers Pleasure Buolamwini and Timnit Gebru learned that 3 commercially out there visible AI devices have been horrible at examining the faces of darker-skinned females. Amazon educated an AI to vet résumés, only to discover it downranked gals.
Nevertheless laptop or computer researchers and numerous AI engineers are now conscious of these bias issues, they’re not generally confident how to deal with them. On top rated of that, neural nets are also “massive black boxes,” says Daniela Rus, a veteran of AI who at the moment runs MIT’s Pc Science and Artificial Intelligence Laboratory. When a neural web is trained, its mechanics are not effortlessly comprehended even by its creator. It is not very clear how it arrives to its conclusions—or how it will fall short.
“For a really very long time, there was this techno-chauvinist enthusiasm that Ok, AI is likely to remedy every single trouble!”
It may not be a difficulty, Rus figures, to count on a black box for a undertaking that isn’t “safety critical.” But what about a better-stakes occupation, like autonomous driving? “It’s essentially fairly remarkable that we could place so substantially believe in and faith in them,” she suggests.
This is where Deep Blue experienced an gain. The aged-school fashion of handcrafted policies may have been brittle, but it was comprehensible. The equipment was complex—but it wasn’t a thriller.
Ironically, that outdated design of programming may possibly stage anything of a comeback as engineers and computer system experts grapple with the limitations of pattern matching.
Language generators, like OpenAI’s GPT-3 or DeepMind’s Gopher, can acquire a couple of sentences you’ve written and maintain on going, producing webpages and pages of plausible-sounding prose. But inspite of some spectacular mimicry, Gopher “still doesn’t truly understand what it is indicating,” Hassabis claims. “Not in a real feeling.”
Similarly, visual AI can make terrible faults when it encounters an edge case. Self-driving cars have slammed into hearth trucks parked on highways, for the reason that in all the tens of millions of hours of video clip they’d been skilled on, they’d under no circumstances encountered that problem. Neural nets have, in their own way, a variation of the “brittleness” issue.