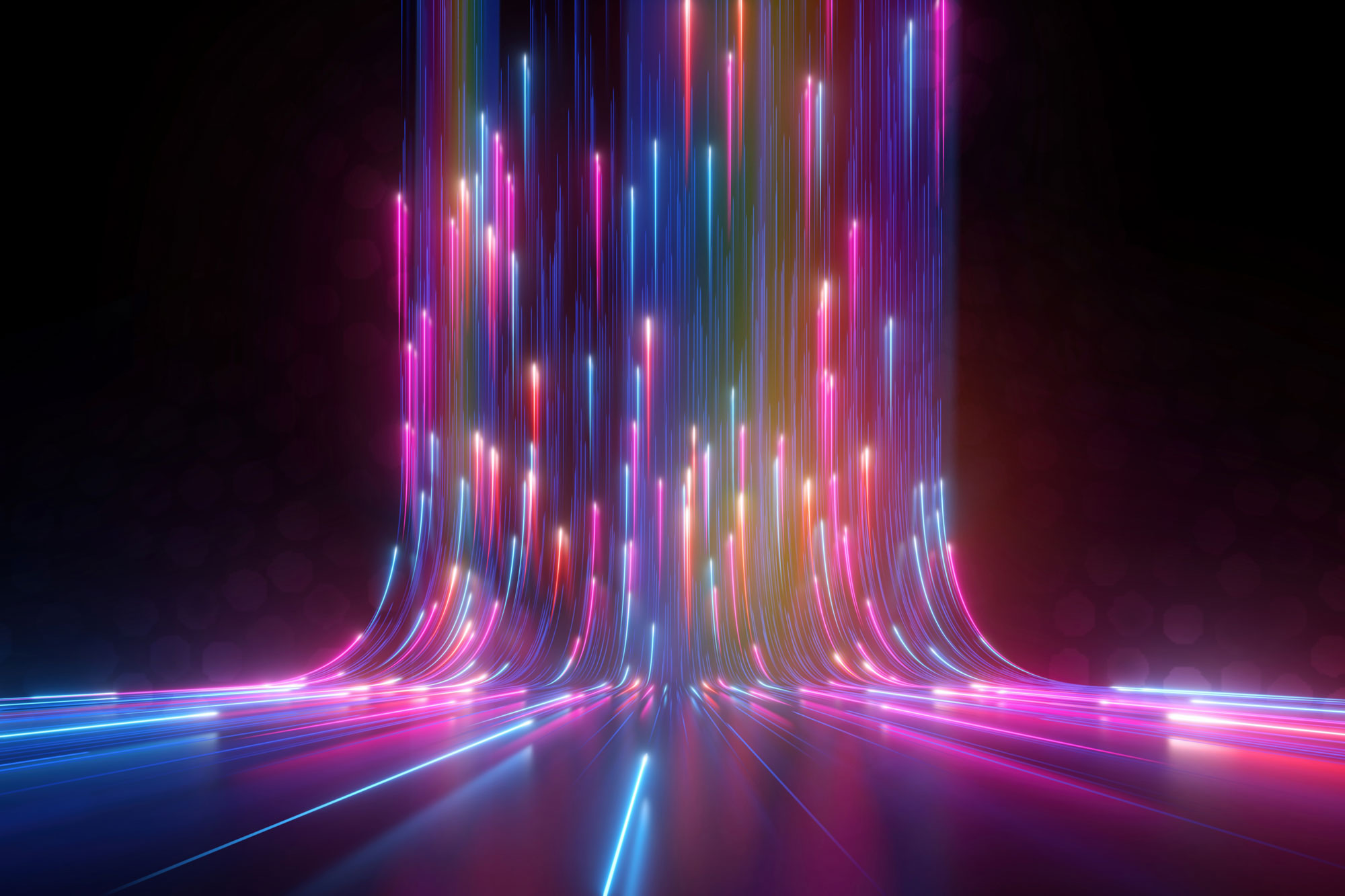
Breaking the scaling limits of analog computing | MIT News
As device-learning designs turn out to be much larger and more elaborate, they require more rapidly and far more strength-effective hardware to accomplish computations. Conventional electronic computer systems are struggling to hold up.
An analog optical neural community could execute the exact same responsibilities as a electronic one particular, such as impression classification or speech recognition, but for the reason that computations are performed utilizing light as an alternative of electrical alerts, optical neural networks can run numerous situations quicker when consuming less energy.
On the other hand, these analog units are inclined to hardware errors that can make computations a lot less precise. Microscopic imperfections in components components are a single trigger of these glitches. In an optical neural network that has many connected elements, mistakes can speedily accumulate.
Even with error-correction procedures, owing to basic properties of the products that make up an optical neural community, some volume of error is unavoidable. A network that is substantial plenty of to be executed in the true planet would be far as well imprecise to be efficient.
MIT researchers have get over this hurdle and identified a way to properly scale an optical neural community. By including a small components part to the optical switches that type the network’s architecture, they can reduce even the uncorrectable glitches that would normally accumulate in the machine.
Their do the job could allow a tremendous-speedy, vitality-productive, analog neural network that can functionality with the same accuracy as a electronic 1. With this approach, as an optical circuit turns into larger, the amount of error in its computations really decreases.
“This is amazing, as it operates counter to the intuition of analog programs, the place greater circuits are intended to have better faults, so that mistakes set a restrict on scalability. This current paper will allow us to address the scalability question of these units with an unambiguous ‘yes,’” claims lead writer Ryan Hamerly, a visiting scientist in the MIT Research Laboratory for Electronics (RLE) and Quantum Photonics Laboratory and senior scientist at NTT Exploration.
Hamerly’s co-authors are graduate university student Saumil Bandyopadhyay and senior creator Dirk Englund, an associate professor in the MIT Section of Electrical Engineering and Laptop or computer Science (EECS), chief of the Quantum Photonics Laboratory, and member of the RLE. The study is printed right now in Character Communications.
Multiplying with light
An optical neural community is composed of lots of connected elements that functionality like reprogrammable, tunable mirrors. These tunable mirrors are known as Mach-Zehnder Inferometers (MZI). Neural network info are encoded into light, which is fired into the optical neural community from a laser.
A normal MZI contains two mirrors and two beam splitters. Light-weight enters the top of an MZI, wherever it is break up into two pieces which interfere with every other just before currently being recombined by the 2nd beam splitter and then reflected out the base to the future MZI in the array. Scientists can leverage the interference of these optical signals to conduct advanced linear algebra functions, regarded as matrix multiplication, which is how neural networks procedure details.
But problems that can occur in each and every MZI speedily accumulate as gentle moves from one particular device to the next. One particular can stay clear of some problems by pinpointing them in progress and tuning the MZIs so before glitches are cancelled out by later equipment in the array.
“It is a pretty simple algorithm if you know what the faults are. But these mistakes are notoriously complicated to verify mainly because you only have obtain to the inputs and outputs of your chip,” claims Hamerly. “This inspired us to glance at regardless of whether it is feasible to produce calibration-free mistake correction.”
Hamerly and his collaborators formerly shown a mathematical method that went a step even more. They could effectively infer the glitches and accurately tune the MZIs appropriately, but even this didn’t remove all the mistake.
Owing to the basic nature of an MZI, there are scenarios in which it is unattainable to tune a unit so all light flows out the base port to the future MZI. If the system loses a fraction of gentle at every single action and the array is really significant, by the conclude there will only be a small bit of energy remaining.
“Even with mistake correction, there is a elementary limit to how good a chip can be. MZIs are bodily not able to know particular settings they need to have to be configured to,” he suggests.
So, the crew made a new sort of MZI. The scientists additional an more beam splitter to the stop of the device, contacting it a 3-MZI simply because it has three beam splitters alternatively of two. Because of to the way this additional beam splitter mixes the light-weight, it gets a lot less difficult for an MZI to achieve the location it demands to send out all mild from out by way of its base port.
Importantly, the supplemental beam splitter is only a couple of micrometers in dimensions and is a passive element, so it doesn’t need any additional wiring. Introducing more beam splitters does not drastically change the size of the chip.
More substantial chip, much less faults
When the researchers done simulations to exam their architecture, they observed that it can get rid of a lot of the uncorrectable error that hampers precision. And as the optical neural community results in being larger sized, the amount of money of error in the system basically drops — the opposite of what happens in a unit with conventional MZIs.
Applying 3-MZIs, they could probably generate a system significant adequate for industrial makes use of with error that has been decreased by a variable of 20, Hamerly says.
The researchers also formulated a variant of the MZI style and design specially for correlated faults. These arise thanks to manufacturing imperfections — if the thickness of a chip is slightly completely wrong, the MZIs may possibly all be off by about the very same quantity, so the problems are all about the very same. They located a way to alter the configuration of an MZI to make it sturdy to these sorts of problems. This approach also enhanced the bandwidth of the optical neural network so it can run 3 moments quicker.
Now that they have showcased these approaches applying simulations, Hamerly and his collaborators strategy to check these approaches on actual physical hardware and proceed driving toward an optical neural community they can efficiently deploy in the actual earth.
This investigate is funded, in aspect, by a Nationwide Science Basis graduate study fellowship and the U.S. Air Pressure Office of Scientific Study.